This is a transcript of the Michael Levin video here: https://www.youtube.com/watch?v=RjD1aLm4Thg
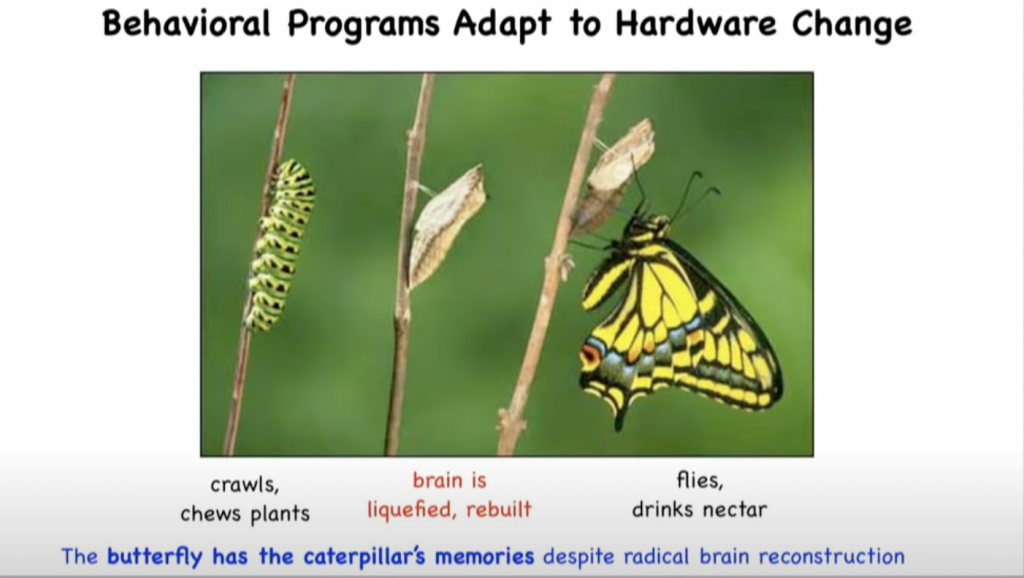
Good afternoon, everyone.
I’m Kristen Grauman.
I’m a researcher at Facebook AI Research and a professor at UT Austin.
And as one of the program chairs for NIRPS this year, I have the pleasure to introduce our next invited speaker, Professor Michael Levin.
Michael’s a professor of biology at Tufts University, and he holds the Vannevar Bush Endowed Chair.
He’s also director of the Allen Discovery Center at Tufts, which examines computation in the medium of living systems.
His academic background is both computer science and biology.
He received his PhD from Harvard Medical School in 1996.
Michael’s group does work at the intersection of developmental biology, cognition, and computational neuroscience.
They are especially interested in applications in regenerative medicine.
And as we’ll see in his talk today, these things can offer inspiration for new machine learning and robotics approaches.
Professor Levin’s research has been recognized with a number of different awards.
I’ll mention just a few.
One is recognition as having made a milestone in developmental biology in the last century from the journal Nature.
And that was for his work on molecular basis of left-right asymmetry.
He’s also received the Established Investigator Award from the American Heart Association and the Scientist of Vision Award from the International Functional Electrical Stimulation Society.
So we’re very excited to hear about his work today.
So please join me in welcoming Professor Michael Levin.
Thank you so much.
And I’m really excited to be able to present this work to a new audience.
And I’m going to give you the main message of my talk right up front.
What I would like to tell you about is that biology has been computing at many scales long before brains came on the scene.
And actually decision-making in all body tissues are mediated by an ancient pre-neural electrical network that is made up of all cells, not just neurons.
And so I think that exploiting this kind of non-neural cognition is an exciting untapped frontier for the development of new robust AI technologies.
And I’m very interested in talking to people about these possibilities.
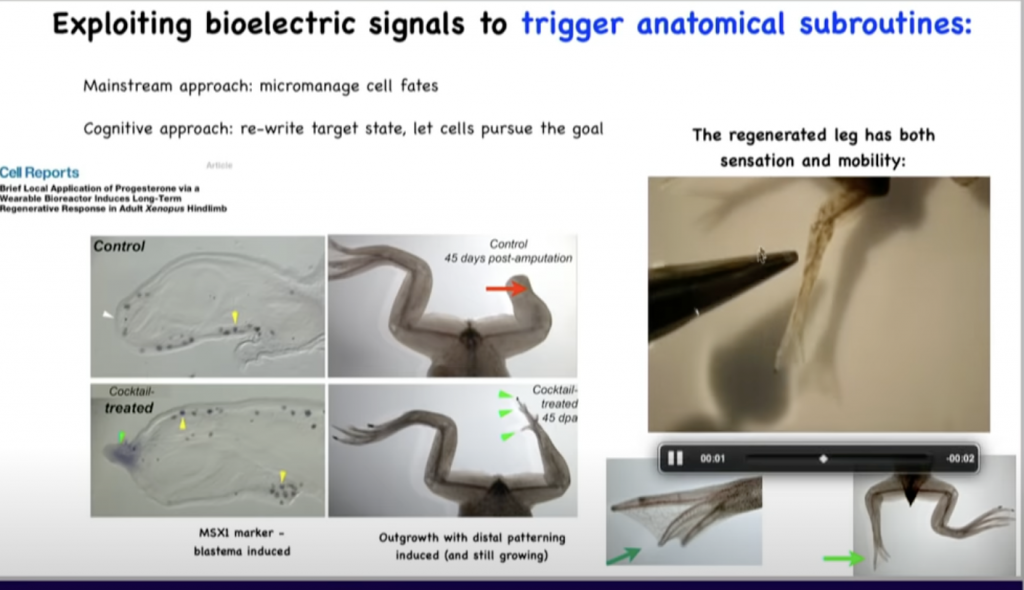
We’re looking for experts in machine learning to collaborate with us to take bioelectrics beyond regenerative medicine and towards many new exciting applications.
So the first thing I would like to do is talk to you a little bit about brain-body plasticity and then to bring to you some examples of somatic cognition in the body, decision-making during the self-editing of complex anatomies.
And then we’ll talk about bioelectric mechanisms that underlie the ability of cells and tissues to remodel towards large-scale goals.
And then I’ll talk about what I think is the future of regenerative medicine and AI and related issues.
So let’s talk about plasticity.
Caterpillars start out life as a soft-bodied robot that crawls and chews plants.
During metamorphosis, its nervous system, including its brain, is basically liquefied.
It’s taken apart and then reassembled into a completely different brain that is able to run a hard robot which flies and drinks nectar.
During this process of complete nervous system being taken apart and putting back together, the memories, in fact, remain.
So things that the caterpillar has learned, the butterfly will still remember.
And this has been well established.
And so it’s very interesting to start to think about how it is that information can be stored in a medium that is undergoing radical deformation.
In work that we’ve done a few years ago, we showed that planaria, these are the flatworms that you see here, they have this amazing property that if you cut off their head or, in fact, any part of their body, they will regenerate it.
They regrow their brain and all the other organs.
So if you train a flatworm to recognize a particular location as being its home environment, and then you amputate the brain, you leave the tail and let it regenerate a new head here, what you discover is that, again, these memories are intact.
So the memory in this animal is in some way imprinted on the new brain that develops after regeneration.
And it challenges us to try to understand how encoding and decoding of complex memories and learned behaviors is able to be propagated across this kind of radical reconstruction of the memory medium.
And, in fact, when we published this a few years ago, actually we weren’t the ones to discover this.
This was first discovered by a guy named McConnell in the 60s.
But people hadn’t really looked at this for years and people got pretty excited, as you can see here on the internet, when we first showed that this was possible.
And going beyond the sort of brain-body interface and the question of how the behavioral programs of the brain interact with the structures of the body, I want to talk to you about this kind of self-editing of anatomy.
There are many different types of cognition, from very simple ones to all the way through the kinds of complex things that we hope to mimic with machine learning and artificial intelligence.
But there are all sorts of living creatures that are able to perform functions that are at various places on this ladder of cognition.
So one example is here, shown by this unicellular creature called the lacrimaria.
If you’re interested in soft-body robotics, take a look at this.
This is a single cell, okay, and this organism handles its physiology, its patterning.
Look at the incredible control it has.
This is real-time, by the way.
This is not sped up.
So look at the amazing control that this has over its structure as it collects bacteria and as it’s basically hunting and eating the different other microorganisms in its environment.
But behavior, structural control, and physiology all in one cell.
There is no brain here as such.
And so unicellular organisms and single cells can be incredibly competent at all sorts of interesting goals.
Now when cells joined to become multicellular bodies, complex anatomical structures, they did not lose this ability to make decisions and to have complex behaviors.
What you’re seeing here on the left is a frog embryo developing, and so these cells cooperate to build a very complex structure.
On the right, you’re seeing the development of a nervous system in zebrafish.
And so all of these cells are now working together to build a structure that’s much larger than the single cell boundary of their immediate surface.
And we need to understand how this works.
All of us started life as a single cell, a single fertilized egg.
And through this process of embryonic development, these complex forms are able to be reliably generated.
So all of these remarkable complex anatomies.
And it’s very important that we understand that this is not just a process that’s going to be solved by stem cell biology, because stem cells are able to generate all of the individual tissues that are needed for an organism.
So what you see here is something called a teratoma.
This is a tumor that has hair and teeth and skin and muscle.
And so all of the stem cell differentiation has gone okay, but what’s missing is the large scale three-dimensional structure.
So one of the important things we need to understand is how do cells decide what they’re going to build and how do they implement that construction.
And so the sort of standard story that people are taught in developmental biology is that there’s this kind of open loop feed forward system where gene regulatory networks give rise to proteins and then through some physics when these proteins interact with each other and the cells perform various functions, there’s this emergent process that give rise to complex anatomy.
But it’s actually much more interesting than that.
First of all, all kinds of embryonic species are highly, have highly regulated development in the sense that if there’s a massive perturbation, they can make up for it.
So for example, here’s an early mammalian embryo.
You can cut it in half and each half will rebuild the other missing half.
This is how you get perfectly normal monozygotic twins.
The opposite is possible too.
You can take two mammalian embryos, mush them together like a snowball here, these mouse embryos, and what you get is a perfectly normal mouse.
We would love to have technology obviously that has this degree of plasticity that can make up for such radical deformations.
Now this is not only an embryonic phenomenon.
The process of regeneration such as the salamanders have is able to rebuild complex large structures during adulthood.
So here’s an axolotl, a salamander that can regenerate its eyes, its jaws, its limbs, its spinal cord, its ovaries, and portions of the brain.
So as an adult, this thing can rebuild very complex structures throughout its life.
The most amazing thing about regeneration is not that it’s able to start, it’s that it knows when to stop.
All of these structures, after the correct anatomical component has been rebuilt, then further growth and remodeling ceases.
And this is very poorly understood, how it knows when to stop.
I want to introduce you to another animal that you’ll see more of later in the talk.
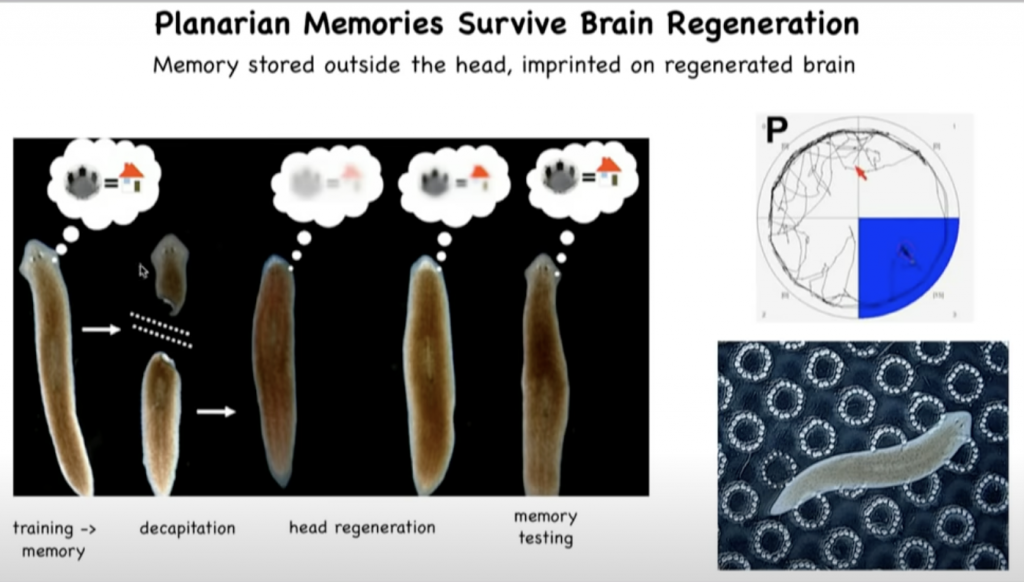
This is a planarian, that same flatworm I mentioned a minute ago.
These can be cut into pieces, the record is 275, and each piece, they’re about 2 centimeters long by the way, and each of these pieces will regrow exactly what’s needed, no more no less, to make a perfect worm.
Every piece of this animal knows exactly what the correct worm looks like, and in fact their regenerative properties are so remarkable that they’ve conquered aging.
These worms are literally immortal.
The individual cells will senesce and die, but the animal as a whole continues to regenerate them, and there is no such thing as an old planarian.
So now, regeneration is not just for so-called lower animals.
The human liver is highly regenerative.
Deer will regrow their antlers, or bone, highly innervated bone, up to a rate of a centimeter and a half per day of new bone growth every year.
And you may or may not know that human children below the age of approximately 7 to 11 are able to regenerate their fingertips, and this was discovered in the 70s.
Then this ability ceases with age, so most of you shouldn’t try this.
So what we see here is that actually the control structure of this process is not an open loop system but actually is a closed loop that has some very important error detection and control functions here, where deviations from this target morphology lead to changes both at the transcription level but also in the physical processes that allow cells to rebuild.
And so there’s this constant homeostatic loop.
And one of the goals of my center is to target the set point, the anatomical set point of this homeostatic process to be able to re-specify what the cells should build.
This is what we’re working towards.
We discovered several years ago that really the capabilities of biological things to build towards a spec are really amazing.
So in the journey from a tadpole to a frog, all kinds of remodeling has to happen.
So the eyes have to move together and forward, the nostrils have to move, the whole face remodels.
And when we made these sort of Picasso-like tadpoles where everything was in the wrong place, the eye was in the back and the jaws were off to the side, the starting positions were all off, they largely still remodeled into a perfectly normal frog.
All of the organs moved in unnatural configurations until they reached this normal frog morphology, even though their starting positions were not the same.
And so we know that what the genome encodes is not a rote set of movements that every organ can simply undergo and give rise to whatever it gives rise to, but they can actually tell when they’re in the wrong configuration and it will keep moving until the correct pattern is constructed.
And understanding this process and taking advantage of it is extremely important.
Over 50 years ago, people realized that if you take a salamander tail and graft it onto the edge of a limb amputation, so you put a tail where the limb ought to be, over the coming months, this structure is actually going to be remodeled from a tail into a limb as this whole system seeks to restore its large-scale global order.
So understand what this means.
This is not an individual cell deciding it’s going to be muscle or skin or anything else.
This is large-scale decision-making at the level of the entire organism.
And so fundamentally, this is a computational problem, and we would like to use this as inspiration for technology that can implement this kind of highly robust plastic behavior.
In the face of radical deformation, very new kinds of environmental perturbations, these systems are able to regain their structure and function.
Now what determines patterning?
We start life as an egg and then a collection of embryonic stem cells, and eventually this is a cross-section taken through a normal human torso.
You can see the amazing invariant locations of all the organs and the geometry of all the structures.
Where does this come from?
DNA, after all, the genome, specifies proteins.
It does not say directly anything about this shape or how it’s edited.
How do cell groups know what to make and when to stop?
And then as engineers, of course, we’d like to know how far can we push this process?
What’s possible to build?
What are the edges of biological possibility?
And so you might be surprised to know that as developmental biologists, we basically don’t know the answer to any of these questions.
We have this significant knowledge gap that while we’re very good at constructing these kinds of networks where we find out which gene products interact with which other gene products, we can’t yet read a genome and find out what shape the resulting organ is going to be.
We can compare it to other genomes where we already know what it looks like, but we can’t read a genome and figure out what geometry it specifies.
What’s even worse in terms of medicine is that if we wanted to fix an anatomical structure here, what we have are molecular information of this kind.
We have these networks that make it very difficult to solve the inverse problem that will allow us to know what to change at this level to make, to have outcomes, desirable outcomes at the system level.
And so we have here huge opportunities for machine learning to impact regenerative medicine beyond drug discovery to help us be able to take this kind of information and understand how it maps to specific anatomy.
In biology, we’re becoming very good at manipulating molecules and cells, the very lowest level hardware that is necessary for the control of complex pattern.
What we don’t really understand yet are the algorithms that are sufficient for the kind of pattern editing examples that I just showed you.
And so can we move biology beyond this kind of machine code?
And I visualize it like this, where computer science has had this wonderful journey from having to alter the hardware when you were programming all the way up to now considerable platform independence where you can really just focus on the control algorithms and the computations.
Biology is still here.
Everything we do in our experiments is rewiring the actual hardware of the organism.
We have not yet gotten to the point where we can focus on the control algorithms and really take advantage of the hardware-software distinction, which I do believe also exists in biology, and this is what my group has been trying to do.
So pattern homeostasis has a number of properties that are, in a certain sense, cognitive.
They show goal-directed behavior towards specific outcomes.
They show flexibility and robustness under variable conditions.
And they allow integration of cell and low-level functions into complex, large-scale outcomes.
And so what I want to talk to you about now are some of the progress that we’ve made about understanding how electrical mechanisms, that is, electrical communication among cells, allow this kind of control.
And basically what I’m going to show you, everything in the next section can be boiled down to one main message, that very much like the brain, somatic tissues form electrical networks that make decisions.
The decisions they make are not about behavior.
They’re about anatomy.
And we now can target the system for some control of large-scale pattern editing.
And so I show you this five-legged frog here to point out that this is not Photoshop.
I’m going to show you a bunch of really strange-looking animals in a minute.
And these are all real, live creatures that live in our lab, because the way that we test our various models of whether we’ve understood how this works is to try to make rational perturbations.
We try to control anatomical decision-making.
So it’s important to realize that brains did not invent their tricks from scratch.
Actually neurons evolved from very ancient cells that were around and performing computations and doing somatic decision-making long before neurons and brains showed up.
And in fact, our unicellular ancestors, before even the origin of multicellularity, already had synaptic machinery, ion channels, neurotransmitters.
This by the way, if you’ve ever wondered why it is that fungi, which are a very distant organism from ourselves, why fungi have compounds in them, like these hallucinogenic mushrooms that are able to affect human cognition, it’s because the basic mechanisms are extremely ancient.
They’re very old.
And that basically in becoming brains, nervous systems speed-optimize functions that were already here long before that.
And so we all know that in the brain you have hardware that’s produced by these ion channels that enable cells in the brain to communicate electrically with each other.
So they mediate electrical state change and can be propagated through a network.
And this kind of hardware gives rise to some very interesting software that we would all like to understand and take advantage of.
So the cognitive and behavioral processing that goes on in the electrical network that is encoded and enabled by this hardware.
Well it turns out that the rest of the body is not very much different.
All cells, not just neurons, have the exact same types of ion channels.
They make electrical synapses to their neighbors.
And they produce electrical path, electrical patterns such as here.
What you’re seeing here is an early frog embryo.
This is sped up.
This is about eight hours of an early frog embryo.
And much like here you can see the electrical activity of the zebrafish brain as the zebrafish processes visual information, what you can see here with pseudocolored are all the electrical conversations that these cells are having with each other as they figure out who’s going to be front, who’s going to be back, left, right, dorsal, ventral, and so on.
So as people try to understand how this, to decode how the electrical states of the brain stand for different memories, behaviors, and so on, we have to understand and we have to crack this bioelectrical code.
How it is that the changing electrical properties of the tissues implement different future anatomical states.
And so here’s an example.
This is just a snapshot of an early frog tadpole like this.
This is the mid-flank.
The different colors are a fluorescent voltage reporter that shows us in living tissue what all the electrical states are.
And each one of these little circles is a single cell.
And this cell has a variety of ion channels just like neurons do that enable them to maintain this particular structure.
So this is what we call a VMEM or a membrane voltage pattern.
And these patterns, we’re able to see them using a variety of technologies such as voltage sensitive fluorescent dyes.
And we do a lot of quantitative simulations to try and understand how the different ion channel and pump proteins give rise to specific patterns in multicellular tissues.
These patterns come in two flavors.
There are normal or endogenous patterns.
So this is again a frog embryo, this time in grayscale.
And you can see here that as it’s putting its face together, here’s basically a pre-pattern or a little map of what the gene expression in the anatomy of the face is gonna look like.
So here’s where the eye’s gonna form.
Here’s where the mouth is gonna be and so on.
So these are patterns that are critical for normal development.
If you move or perturb these patterns, the anatomy will be altered.
These patterns can also be pathological.
Here you see the earliest signs of cancer.
We induced a tumor in this tadpole.
But before the tumor shows up, these cells right here, you can already see their abnormal electrical state as they stop communicating with their neighbors and sort of revert back to an almost unicellular-like existence and become cancer cells.
So functionally, we’ve developed some tools to control this type of signaling.
In this non-neural cell network, basically we do not apply electrical fields.
That’s not how this is done.
There’s no electrical electrodes being used here.
What we do is we manipulate the endogenous ion channels that allow cells to produce electrical states or we perturb the connectivity.
So we can either control the topology of the network or actually the electrical state.
And we use a variety of genetic, pharmacological, optogenetic, and those kinds of techniques.
So I want to show you some examples of why this is important, what you can do when you alter the electrical circuits.
So one thing you can do is you can convince cell groups to become different organs.
And I don’t mean to control individual cell types.
This is not about stem cell differentiation.
But for example, we can take a region of this gut.
So this whole thing is the gut of this teple.
We can take this region.
We can change its electrical properties by opening a particular kind of channel, this voltage-gated potassium channel.
And what will happen is this whole, once we establish a particular electrical state, this whole region becomes an eye.
And if you section this eye, you will see all the same interior components that you would find in a normal eye.
So retina, optic nerve lens, all of these things are in there.
So this is a modular control that says, right here, you’re going to form an eye.
The same thing can happen in planaria, where if we cut off the head and the tail, and we take this middle fragment, which has this very interesting head-tail electrical pre-pattern, what we can do is take these cells and convince them that they should make a head instead of a tail.
And you see that here.
This is a two-headed worm.
In fact, you could go in the other direction.
You could make a no-headed worm.
And in all cases, this is very important.
This is modular.
Because what we’re not doing is going in and micromanaging the process.
We are not trying to lay out cell by cell, as you might if you were doing bioengineering.
We’re not trying to lay out cell by cell what the structure is.
We’re providing, actually, a fairly small amount of information that tells this region what it should be.
It’s basically a subroutine trigger at that point.
And so using this process, some of the other things you can do, we can take this flatworm that has a triangular head.
And when we cut off the head, we can treat the remaining fragment in a way that convinces the new cells to build heads that belong to other species of flatworms.
So here’s a nice flathead like this P. falina.
Or you can make a round head like an S. mediterranea, or a triangular head like the original should be.
Notice that, first of all, it’s about 150 million years of evolution that separate these animals.
So you’re making heads with brain structures and stem cell distributions that belong to these other species across about 150 million years of evolution.
Also note that the genome here is normal.
We have not done any genomic editing.
This is not about changing the genome.
This is purely a physiological change.
This is interacting with the real-time software, as it were, of the physiological system, not rewiring the genes or the protein networks that are existing in this animal.
So all of these animals with heads belonging to other species have a perfectly normal wild-type genomic sequence that belongs to Deghizia dorata cephala.
So you can go further than this, and you can reach regions of the planarian morphospace that evolution isn’t using.
So from a normal flatworm, you can make things like this that are sort of spiky.
You can make these that look like hats.
They’re three-dimensional.
They have an interior compartment.
Or you can make ones that look like this with tubes coming out of their backs.
So we’re just now beginning to really understand the way that different anatomical structures are encoded in the electrical properties of the network.
But it’s very clear that there’s a very tractable, profoundly important level of software control that can be implemented beyond the genome.
All of these animals have the same genomic sequence.
And so this is really important to begin to learn how to target this for various applications.
We’ve done lots of computational modeling to try to understand these electrical circuits and how the dynamical systems properties of these circuits map onto specific anatomical outcomes.
And so one of the predictions of this kind of unusual view that these are all – that these pattern memories are states of physiological electrical network is that we ought to be able to rewrite this pattern memory.
And I want to show you one example that we just published last year, which is that if we take these normal-looking planaria – so one head, one tail – and in fact, molecularly, if we look at the markers, so the head markers are in the head.
There are no head markers in the tail, and so on.
These will give rise to a one-headed worm if we chop off the head and the tail.
These will give rise to a two-headed worm.
Why would an animal that has a perfectly normal one-headed anatomy and apparently normal molecular expression pattern, why would they give rise to two-headed worms?
And the reason – and again, this is not anything to do with genomic editing.
And the reason is because what we did was we went in and we altered the electrical pre-pattern that dictates what this tissue is going to do if it is injured in the future.
So this one-headed animal is bearing an electrical memory that says that if I get injured in the future, what I’m going to do is build two heads instead of one.
So the electrical pattern is not a readout of what the anatomy is now.
It is a piece of encoded information, a patterning engram that is used if the animal is injured, and it is used to guide morphogenesis in the future.
So what we see is that this body, a single body like this, can store at least one of two different pattern memories.
It can store one-headed or a two-headed pattern memory.
No doubt probably more.
We’ve only been able to access two so far.
Now this is really a very important example because it is not just about changing the way that regeneration progresses after the injury.
This is a long-term phenomenon.
And by that, what I mean is that if we take this two-headed worm and – so we start with a one-headed worm, we amputate the head and the tail, we perturb the electrical network, you get a two-headed worm.
And now we take this two-headed worm and we leave him alone for a couple of weeks in plain old water, so no more manipulation of any kind.
We amputate the original head, we amputate this weird ectopic posterior head, and we ask what’s going to happen.
And when we first did this experiment, of course, the standard paradigm said that, well, the genomics of the worm are normal, and you’re going to cut off this ectopic head, so of course the rest of it is just going to go back to normal, and you’re going to get this normal one-headed worm, right?
And of course, I’m showing it to you because that’s not at all what happens.
What happens is that in perpetuity, once you’ve shifted that electrical network, that pattern memory has now been shifted to a two-headed state, it is now permanently two-headed.
So if you keep cutting this animal again and again with no further manipulations, it will continue to regenerate to that new specification of what a worm should look like.
And you can, by the way, we have now ways to take it back and take a two-headed worm and remodel it back to one head.
So this is very interesting.
This has a number of key properties of memory.
It’s long-term stable.
It’s rewritable, or labile.
It has latency or conditional recall, as I just showed you.
We can do it so that nothing happens until the animal needs to recall it from injury.
And it has discrete possible outcomes, one-headed or two-headed.
So what we’ve been doing is trying to develop techniques to basically extend certain connectionist models to this, be able to help us guide these experiments where stable attractors in the state space of these electrical circuits correspond to specific downstream anatomies, of course, mediated by all the different genes that they turn on and off.
So this is really critical for us now is to understand the large-scale properties of this bioelectric code and how these pattern memories are literally encoded in the stable states of tissues.
It’s important not only for the basic science of it, but for applications in regenerative medicine.
So unlike salamanders, frogs do not normally regenerate their legs.
So they either grow nothing or a very thin cartilaginous spike when you amputate the leg.
And what we’ve been able to do is to come up with a cocktail that alters the bioelectric state of the cells at the wound to convince them to regenerate a new leg.
So here you can see in 45 days it’s starting to make some toes.
Here’s a toenail.
And then eventually it’s a pretty good leg that can feel, touch, and it’s both sensitive and motile, so the animal can use these legs.
And then eventually you get some really nice patterning, and then you have to wait for it to grow.
And so the idea is that this is the first steps towards a program for regenerative medicine, where we will be able to induce cells and tissues to regrow various complex organs.
This does not require implantation of stem cells.
It does not require genomic editing.
This is purely about triggering the kinds of cascades, the kinds of patterning subroutines that these animals already have embedded in their electrical software.
And of course, next after this frog is mammalian application, so we’re trying to work up to human medicine at some point.
So one of the other things we’ve been able to show is to use some of our predictive simulations of the bioelectrical signaling to reverse birth defects of different types.
So here’s what a tadpole brain, a normal tadpole brain, should look like.
You see here the forebrain, the midbrain, and the hindbrain.
And under the exposure to various teratogens, and we’re talking about things like alcohol, nicotine, various other nasty chemicals, or even mutations in really important genes such as Notch, what you get are severe brain defects.
So the forebrain is basically missing, the midbrain is a bubble, the hindbrain’s all messed up.
And what we’ve been able to do is to produce bioelectrical simulations that tell us what electrical state we would have to restore in order to get back a normal brain.
And in fact, from these simulations, we can extract information for candidate drugs that will open and close various ion channels.
And you can see here a successful applications of this.
This animal has a mutation, it’s expressing a mutation in a gene called Notch, which normally causes this terrible malformation of the brain.
But the structure, the gene expression, and even the function of the brain.
So we gave them IQ tests, believe it or not you can do that in tadpoles, you can test them for learning and memory.
And they get their memories back, despite this really terrible genetic mutation.
So we really think that this bioelectrical network is an incredibly tractable layer that allows us to intervene in the default state that is encoded by the genome.
And so in the future, what we would like to do, this is kind of the endgame for this field which is a very long way away, but the idea is to have a kind of biological compiler where we would be able to design our creatures the way people now design things in CAD or for 3D printing.
Where you can design at the level of anatomy and then we would understand well enough what you would have to do to make this structure actually grow.
And evolution learned very early on to exploit the computational properties of electrical circuits.
Electrical circuits have some very convenient properties in terms of information processing and evolution caught on to this very early.
And so cracking this bioelectrical code will have impacts not only on regenerative medicine, but also in synthetic bioengineering, morphological computation, and so on.
This is really I think a very important emerging field.
And so what I want to close now with is to talk a little bit about the future and this idea that perhaps we could make a highly robust machine learning platform technology that is based on non-neural architectures.
And we really are interested in collaborators for this.
Is it possible that some of the limitations of current machine learning is because people have really been focusing on imitating brains and especially human brain architectures?
And what would happen if we stepped way back and based our attempt at this on cells and tissues that are doing much more fundamental information processing than what we see in brains?
So, in fact, some people have started looking at this in other structures.
So bone, heart, pancreatic cells, all of these cells have been shown to exhibit aspects of learning and in particular robust adjustment to novel conditions.
One of the most amazing things about living things is how good they are at adapting to novel circumstances that they’ve never seen before.
And cracking this problem will be very important for robust robotics and artificial intelligence, but it’s critical to note that this is already present in cells long before the animals acquired brains.
So, one of the things that we’re doing is to try to extend the basic results of connectionism, neural networks, and all of those kinds of things to beyond the brain.
Try to develop a kind of mathematics and training algorithms that can be used on non-neural networks.
And the other thing that we’re doing is we’ve had a little bit of success coming up with contributions to what’s called the robot scientist field, which is basically platforms that use machine learning to help us actually understand what’s going on and to discover not just to do number crunching, but actually discover models of the kinds of systems that we’re working with.
And so what I would like to do now is to thank all the people that have done some of the work that I’ve shown you today.
I want to thank my funders, and particularly the Paul G. Allen Frontiers Group and DARPA.
And if you wanted to see what two-headed worms actually look like and behave like, this is what it is.
Here, you see them.
And we now, we actually have openings both for postdoctoral work and for visiting scientists.
So if anybody’s interested in making robotic bodies for various biological systems, new AI platforms based on non-neural architectures, machine learning, and all kinds of computer science applications, we would love to collaborate.
And you can get in touch with me here, and I will take questions.
Thank you.
Hey.
How do you determine which electrical activity is just due to functional movement and things like this in the organism’s day-to-day life, and which aspects are going to be used for something like regeneration?
And then how do you actually edit it?
Yeah.
So, okay.
So the convenient thing is that the kinds of electrical states that are important for decision-making at the anatomical level have a very different time scale than the kinds of things that guide behavior.
So anything that has to do with muscle motion, with neural activity, the time scale you’re talking about is milliseconds.
The electrical activity that we deal with, these patterns are stable, and they change over a scale of minutes, hours, in some cases, days.
So it’s an entirely different set of, if you will, frequencies that you deal with.
And the way we, I think your second question was how do we edit it?
Yeah.
So there’s a couple of different approaches that we take.
In our model systems that we use at the bench, the best way to edit it is to knock in controllable ion channels.
So we will put in novel, maybe light-gated, maybe drug-gated, different ion channels that allow us to have very fine control over all the electrical properties.
In biomedical applications, this kind of gene therapy is probably best avoided.
So what we do there is to develop cocktails of drugs that will open and close specific ion channels that are already in the patient.
So we have a variety of computational tools that let us look at what channels and pumps are expressed in the tissue of interest, and then try to come up with a strategy that’s going to get us to the electrical signaling that we want.
Does that interfere with the short-term electrical signaling?
It really doesn’t.
One of the really remarkable things that we find…
So I should point out that back in about 2000, when we first suggested this kind of idea, this was considered completely crazy.
People said that electrical properties of cells are housekeeping parameters, and that if we mess with it, everything’s going to die, that you’re going to get an uninterpretable toxicity.
None of that is true.
It turns out that cells are very, very good at distinguishing the different types of electrical signals.
And things that we do at the long-term scale do not affect behavior.
They can, of course.
You can overdo it.
I mean, part of what we’ve been doing for the last 15 years is coming up with protocols that have the least amount of side effects possible, right?
So you certainly can overwhelm the system.
But these are designed to work in parallel.
And in fact, in all of these organisms, the regenerating planarian or the regenerating salamander is running around, eating, behaving, doing all that stuff, while all the cells are figuring out what to do.
So there’s a multi-scale set of problem solving that does not interfere with each other.
It’s a phenomenal talk.
Thank you.
Thank you.
So, okay.
Thank you very much for a very interesting talk.
Actually, I was very excited about your biological-inspired work.
And I think there is a research area called synthetic biology, and then people are trying to create…
Actually, people can create artificial cells from scratch.
And also, now, if you attach electrodes to the small insects, you can control the insects by sending a signal to them.
And also, people are trying to create truly intelligent robots from robotics perspective and also algorithmic perspective.
So I’d like to know your personal opinion about what is the correct direction of creating truly intelligent agent or creatures or whatever.
I mean…
Yeah.
Well, I can say a couple of things.
We do have a section of my group that works on synthetic morphology.
So we have some really promising…
It’s not published yet, so I didn’t bring it up here.
But we have some pretty amazing new data on the ability of cells to learn to cooperate in completely different configurations.
So you can make artificial living machines that are nothing like the animal from which they came.
And they’re able to learn to cooperate.
And through some sort of a combination of emergence and guided self-assembly, we can make some machines that are just amazing.
I think that’s a very exciting field.
Of course, the other thing that is really interesting is to try to extract some of the policies that cells and tissues use and try to implement them either directly in the hardware or to bio-interface.
So we’ve done a little bit of that too, is try to interface some…
One of the things I didn’t bring up here for lack of time is we found out that if you make a tadpole where the eyes are on the tail instead of in the head, those tadpoles can see out of those eyes perfectly well.
The brain has…
For millions of years, it’s evolved to expect visual input from this region up in the head.
And all of a sudden now, there’s this weird itchy patch of tissue in the back that’s sort of sending you some signals.
The brain has no trouble figuring out that that’s visual data and being able to learn.
So this plasticity is incredible.
And we need to understand how this works.
And then I think the artifacts that we make are going to be just transformative.
Fascinating.
Thank you very much.
Great talk.
Thank you.
Do we know something about plants in terms of electrical signaling?
And also there is mechanical signaling, which is very relatively slow.
So do you know anything about interactions between these different modes?
Yeah, great questions.
So plants, yes.
My group doesn’t work on plants, but there’s a couple of other labs that do.
And it’s very interesting.
Plants do very similar things with electrical signaling as a lot of what I showed you here is true in plants as well, which is remarkable because plants and animals share different origins of multicellularity.
So they both discovered this independently.
And that’s because the ancestors, even from bacteria, so even bacterial biofilms propagate potassium waves that are brain-like.
And there’s been some interesting work on that.
So the decision making, for example, in plant roots as they go through the soil and figure out where they’re going to go, all the way through morphogenesis of the plant itself, a lot of what I’ve said here holds for plants as well.
Mechanical forces, yeah.
Mechanical forces are absolutely also important in all of this.
And mechanics interacts tightly with bioelectrics.
There are stretch and pressure sensitive ion channels.
So they will convert mechanical forces into electrical forces.
And there are proteins that do the reverse, that basically under electrical gradients across the membrane, they will exert stress and strain.
So those systems interact.
Also, of course, both of them interact with more familiar genetic and biochemical signaling.
And so these are three pieces of the puzzle.
And the whole system is kind of this dynamical system where all of these things constantly interact.
The one question that we always ask ourselves is, at what point in that control structure is it most efficient to intervene?
So right now, the majority of my colleagues are working on biochemistry and genetics.
So they’re intervening at the molecular level.
So they’re looking for growth factors and diffusible signals.
What we have seen is that intervening in the electrical level gives extremely efficient control.
So if you want an eye, you can try to assemble it cell by cell.
But it’s really much more efficient to trigger the whole subroutine, as it were.
And so I think that a lot of the important decisions are made at the electrical level.
But it’s absolutely true that mechanical forces and transcriptional and chemical networks are downstream as part of how this stuff gets executed in the actual tissue.
Hi.
Thank you for your talk.
It was very fascinating.
So you talked a lot about how on cells that regenerate limbs are making some sort of decision.
And you said that plant roots, they make decisions as they navigate through the ground.
And somewhere on a higher level, we also make decisions in our brain.
So somewhere between there, this thing called consciousness arises.
Is there any sort of real definition of consciousness?
And if so, is it a gradient?
Is it a line?
Can you speak to that at all?
Yeah.
I get into enough trouble talking about decision making at the level of cells.
So I don’t think I’m going to voice an opinion on consciousness per se, because there’s a lot of people that work on this and argue about that stuff.
But I will say that there is an emerging field of basal cognition.
And these are groups of people that talk about memory learning, sensing, creativity, all the kinds of things, all the way from bacteria, plants, animals, cells, and in fact, even group colonial organisms like anthills.
There are things that an anthill knows that the individual ants don’t know and so on.
So there’s lots of people that worry about that kind of stuff.
And we discuss all these things at meetings.
So I don’t think I have anything profound to say specifically about consciousness.
But I do feel very strongly that all of this is a continuum, that there is nothing radically special about it.
There’s a lot of people that feel that some of these terms should be left sort of exclusively in the realm of human psychology.
And I think that’s a profound mistake.
I think that’s a mistake both on evolutionary grounds and just there is no principle distinction.
There are only shades of quantitative difference.
And so you can see things like anticipation.
Yeast can anticipate.
Yeast can learn simple patterns and they’ll anticipate to them.
These things go all the way down to the very base of the tree of life.
And so what you’re going to call as consciousness, I mean, I have a very good colleague who thinks that consciousness appears when something she calls UAL, unlimited associative learning, takes place.
And there are only some organisms that are able to carry out a kind of generic associative learning and some organisms cannot.
And that’s where she puts the line.
I’m not sure.
I think it’s as reasonable as anything else.
But for sure it’s a continuum.
And whether it’s consciousness or just functional cognitive abilities, they go all the way to the beginning.
Thank you very much.
I was amazed by your talk.
When you apply these frequencies or this electronical signal, how do you do that?
Do you put in just like an electrode into something or are you poking it with something?
And what is the timeframe that these signals need to be applied?
Right.
Timeframe.
That’s an important question.
So we never apply any electrical fields.
We have no electrodes.
We apply no electrical fields and we don’t do anything with frequencies.
What we try to do is to alter the endogenous state of resting potential across cells.
And the way we do that is we target the ion channel proteins that are endogenously expressed in these cells.
We either close them, we open them, and we thus affect the electrical state.
So this is done either genetically or pharmacologically in the case of our regenerative medicine applications is with drugs, small molecule drugs.
But we can in, let’s say in tadpoles, a really nice way to do it is optogenetics.
You put in something like channelrhodopsin and then you shine a light and the pattern of the light can tell, you can really have pretty fine control over where the electrical states go.
The timescale is really critical because a lot of the types of effects that I’ve shown you are literally triggers to these, what we call these subroutine calls, right, of patterning.
The time that you have to do it is incredibly short.
So in the frog leg, we treat the wound, we treat the amputation wound for 24 hours.
That gives you nine months of leg growth.
So after the first 24 hours, you walk away, you never touch it again, and the whole thing goes for 24 hours.
You could intervene at different steps of the way.
If instead of a frog leg, you wanted something else, you could go in and try to make it do something else.
So we’re certainly working on that as a way to understand what’s happening.
But the treatments are often incredibly brief because one of the properties of these electrical circuits is they have these stable attractors that once you knock them into that sort of neighborhood of the state space, you don’t need to hold them there.
They’re going to maintain state.
And so our goal is to map out all of these things to understand what is the minimum we can do to get the maximum outcome.
Thank you.
Hi.
Could you talk more about these new architectures, these non-neural architectures, and what would that get us?
Would we have more compositional systems, more flexible ones, things that don’t just think but also feel, or even like a test bed for designing new organisms or new, like, use that to design biology?
Yeah, I think what it’s going to get us, I don’t know if it’s going to get us systems that feel and how we would know if they did.
That’s a whole other sort of kettle of fish.
But what I think it’s going to get us is much more robust, adaptive systems.
One of the things that biological systems are really good at is adaptively adjusting to very novel environments.
And so I think one of the problems that we have in this field, and you guys can correct me if I’m wrong, but I think that one of the problems we have is that systems that are very good at one particular task often are not that great when you shift it to a different task.
Biology isn’t like that.
These cells and these tissues are incredibly good at dealing with very novel scenarios in an adaptive way.
And one of the differences between biological structures and the kinds of artifacts that I’ve seen people make is that biology has competency at every level.
So the individual molecular networks have memory and flexibility.
The cells do, the tissues do, the organs do.
Every level has its own competency.
So you’re building things out of very intelligent components.
And there are various other things that we can talk about at length.
But I think what it’s going to get us is systems that are fundamentally good at pursuing goals in the light of an unfriendly environment.
That’s what all of life is, right?
These biological systems have very specific goals.
The environment throws all kinds of crazy scenarios at them.
And they are really good at navigating all of that.
And I think we don’t really know how to do that.
So that’s what we’ll get.
And one more.
Do you have any sketch of how we would build such a thing?
I have many ideas on how we should try.
And so I’m happy to talk to you afterwards and talk details.
Thank you.
Hi.
I found this is an absolutely incredible talk.
And one thing that came to mind when I was thinking about this talk was ethical concerns.
It seems like when you’re working with small animals, it doesn’t really matter.
But when you start getting into humans and start doing things that could potentially have really systematic effects on them, that people may start objecting to that.
And I’m wondering if you have thoughts about how to head off those ethical concerns.
Sure.
Yeah.
Good question.
I can say a couple of things.
I think that on the one hand, I’ll point out that in terms of ethical concerns, the kinds of things that I’ve been talking about here are way low on the list of compared to competing technologies that are already out there.
So people that are doing genomic editing, people that are doing infectious disease research, you know, creating novel viruses.
And I mean, the stuff that’s being done is like, you know, way beyond anything we’re talking about here.
And in fact, on that scale, this is actually fairly subtle.
Because what I’m specifically saying is I think we can develop a strategy for regenerative medicine that does not require manipulating the genome.
It does not require any kind of viral delivery vectors or all this.
So I think there are way bigger problems in the field than what we’re doing in terms of ethics.
All right.
Well, thanks very much.
Hi.
So the ML community has done some work in mimicking signal transduction networks, particularly in T cells or immune cells in general.
And so I’m curious if you can help me understand what the relationship between some of these ion channel based networks and signal transduction networks are and how that kind of ties into the AI theme.
And secondly, so the computational systems biology community has kind of developed a lot of computational models of this type of thing.
So ranging from ODE based networks to partial differential equations to like Boolean networks and trying to scale those up to a whole cell models.
Does that relate to what you’re talking about here in terms of this AI platform for non-neural architectures?
Is there a relationship?
Are you doing any of that in your lab?
Yeah.
So that’s related.
That’s actually a very kind of lengthy question.
It sounds like we should take that up.
Okay.
Yeah.
I think we can talk about that at length.
That’ll take a pretty lengthy answer.
But I’m very happy to talk to you about it.
Hi.
A related question.
There is a long history of reaction diffusion models for patterning.
And so I was wondering if the mechanism you, and to some extent it has been confirmed with BMP signaling in limbons.
So I was wondering what is your view of the interaction of this electrical system with a reaction diffusion system?
Is it upstream?
Is it interfering with it?
Is it the reaction diffusion system itself?
Yeah.
So two things.
As far as we can tell right now in the cases that we’ve looked at, it is upstream.
So it helps control the downstream RD systems for chemical morphogens.
And as far as, what was the other, sorry, what was the second question?
I was wondering if it could be the reaction diffusion system by itself.
Yeah.
Yeah.
Actually, that’s true too.
So one of the really interesting things about these kind of electrical networks is that when they’re spatialized and distributed over a group of cells, they themselves purely at the electrical level with no transcriptional changes underneath can do spontaneous symmetry breaking and Turing-like patterns.
So that’s absolutely true as well.
Okay.
Thank you.
Thank you.
Thank you.
Thank you everyone for all the great questions and thank Michael Levin.
Thank you, Kevin.